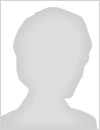
This will count as one of your downloads.
You will have access to both the presentation and article (if available).
In contrast to conventional methods, the proposed methodology incorporates an advanced image enhancement algorithm. This algorithm involves a multi-step preprocessing approach, including applying a Kuwahara filter, a median filter, and a homomorphic sharpening filter. The primary purpose is to enhance the visibility of tumor structures in MRI images, effectively highlighting relevant features while mitigating unwanted details and noise.
This study adopts a comparative analysis strategy, independently employing VGG16, ResNet18, and DenseNet pretrained models to classify brain tumors. By evaluating and contrasting the performance of these models, we aim to identify the most adequate one in terms of accuracy and efficiency. Our findings demonstrate that implementing this methodology with deep learning architectures results in an outstanding classification accuracy of up to 95%.
This research brings significant progress to automated brain tumor classification by providing detailed insights into the strengths and limitations of various deep-learning models used in medical imaging. The results serve as a practical guide for future research, which could lead to the development of more accurate diagnostic tools. Specifically, the findings can help create targeted tools for diagnosing glioma, meningioma, no tumor, and pituitary classes, which could bring advancements in medical imaging technology, leading to more efficient neurology diagnostics.
This study investigates the integration of Artificial Intelligence (AI) to streamline the analysis of OCT images. Employing Deep Learning (DL) models—VGG16, ResNet18, DenseNet—transfer learning, and data augmentation, the research aims to enhance OCT images, optimize disease recognition, and accurately classify CNV (Choroidal Neovascularization), DME (Diabetic Macular Edema), DRUSEN, and NORMAL pathologies.
The dataset undergoes preprocessing, resizing, and enhancement to refine the images. The DenseNet model achieved the highest test accuracy of 92.41% after 25 epochs, demonstrating its potential in efficiently diagnosing ocular pathologies through OCT images.
View contact details
No SPIE Account? Create one