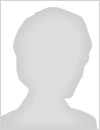
Artificial intelligence (AI)-based reconstruction is a promising method for MRI reconstruction. However, deep neural networks may exhibit instabilities in conditions that are difficult to identify with patient images. The purpose of this work is to investigate whether digital phantoms can help evaluate the performance of AI-based MRI image reconstruction. We chose AUTOMAP as an example of AI-based reconstruction method, with the network being trained with 50,000 paired patches of T1W healthy brain images and corresponding noisy k-space data. We tested the network with noisy k-space brain images, digital phantom images, and hybrid images (i.e., brain test images that contained an inserted lesion-like object). The set of brain test images was used to evaluate the global reconstruction accuracy in terms of mean squared error (MSE). The digital phantoms were designed to test image homogeneity and resolution. The hybrid images were constructed to mimic unhealthy patient for testing whether the AI reconstruction model trained with all healthy brain images would yield equal performance on abnormal brain test images. We also selected two test cases (one brain and one phantom) to quantitatively compare AI-based reconstruction and IFFT in terms of local reconstruction accuracy, which was measured by mean intensity and homogeneity of a region of interest (ROI) in a range of noise levels.
It was observed that AUTOMAP reduced noise variance on brain images within our pre-trained noise range compared to the IFFT reconstruction, but increased variance on phantoms creating inhomogeneous appearance in reconstructed phantom images. In hybrid images, similar degradation of performance was shown in the lesion-like area. Our preliminary results demonstrated that performance of the neural network was highly dependent on the training dataset. If the training data only includes healthy subjects, reconstruction of pathology regions may not be as good as healthy anatomic regions. Digital phantoms helped identify this potential generalizability issue in this AI-based MRI reconstruction.We evaluate the Pre-Whitening Matched Filter (PWMF), “Eye-Filtered” Non-Pre-Whitening (NPWE) and Sparse-Channelized Difference-of-Gaussian (SDOG) models for predictive performance, and we compare various training and testing regimens. These include “training” by using reported values from the literature, training and testing on the same set of experimental conditions, and training and testing on different sets of experimental conditions. Of this latter category, we use both leave-one-condition-out for training and testing as well as a leave-one-factor-out strategy, where all conditions with a given factor level are withheld for testing. Our approach may be considered a fixed-reader approach, since we use all available readers for both training and testing.
Our results show that training models improves predictive accuracy in these tasks, with predictive errors dropping by a factor of two or more in absolute deviation. However, the fitted models are not fully capturing the effects apodization and other factors in these tasks.
View contact details