The measurement of the minimum temperature difference (MTD) at which a four-bar target can be resolved is an important task for assessing the performance of infrared imaging equipment. Recently, there have been efforts to use an automatic objective approach based on machine learning to replace the classical manual subjective MTD measurement approach, which is heavily influenced by the psychological subjective factors of the operator and is limited in accuracy and processing speed. However, the scale variability of the four-bar targets and the high intrinsic target-background similarity in infrared images have been observed to present a challenging problem for automatic MTD measurement. We present a concealed multiscale feature extraction network (CMF-Net), a backbone for automatic multiscale four-bar target detections, in an attempt to address the aforementioned challenges. We first propose a multilevel feature pyramid module to construct a robust representation of multiscale four-bar targets. Next, to distinguish between the four-bar targets from the background, a target saliency enhancement module (TSE) is introduced to capture discriminative features about the target by encoding local and global contextual information. Experiments show that the proposed method is effective and achieves state-of-the-art four-bar target detection results on infrared-image dataset compared with existing methods. |
ACCESS THE FULL ARTICLE
No SPIE Account? Create one
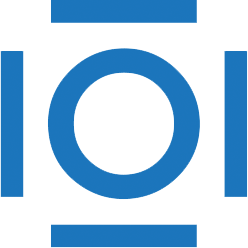
CITATIONS
Cited by 1 scholarly publication.
Target detection
Feature extraction
Infrared imaging
Infrared radiation
Infrared detectors
Minimum resolvable temperature difference
Lithium