|
ACCESS THE FULL ARTICLE
No SPIE Account? Create one
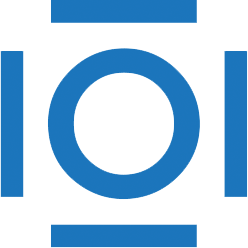
CITATIONS
Cited by 5 scholarly publications.
Neural networks
Algorithms
Stochastic processes
Neurons
Interference (communication)
Data modeling
Evolutionary algorithms