Purpose: Due to the high incidence and mortality rates of lung cancer worldwide, early detection of a precancerous lesion is essential. Low-dose computed tomography is a commonly used technique for screening, diagnosis, and prognosis of non-small-cell lung cancer. Recently, convolutional neural networks (CNN) had shown great potential in lung nodule classification. Clinical information (family history, gender, and smoking history) together with nodule size provide information about lung cancer risk. Large nodules have greater risk than small nodules. Approach: A subset of cases from the National Lung Screening Trial was chosen as a dataset in our study. We divided the nodules into large and small nodules based on different clinical guideline thresholds and then analyzed the groups individually. Similarly, we also analyzed clinical features by dividing them into groups. CNNs were designed and trained over each of these groups individually. To our knowledge, this is the first study to incorporate nodule size and clinical features for classification using CNN. We further made a hybrid model using an ensemble with the CNN models of clinical and size information to enhance malignancy prediction. Results: From our study, we obtained 0.9 AUC and 83.12% accuracy, which was a significant improvement over our previous best results. Conclusions: In conclusion, we found that dividing the nodules by size and clinical information for building predictive models resulted in improved malignancy predictions. Our analysis also showed that appropriately integrating clinical information and size groups could further improve risk prediction. |
ACCESS THE FULL ARTICLE
No SPIE Account? Create one
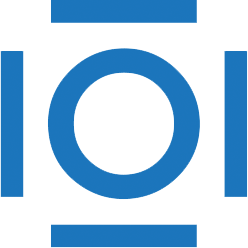
CITATIONS
Cited by 3 scholarly publications.
Lung cancer
Lung
Convolutional neural networks
Cancer
Data modeling
Tumor growth modeling
Image segmentation