It is often said that the practice of medicine is both art and science. The interpretation of medical images (e.g., radiology, pathology, cardiology, telemedicine) is no exception and perhaps is even the paragon of this duality. At one level the science part seems obvious—the functioning and capabilities of the human visual system are well known and characterized, as are the basic characteristics, features, and appearance of lesions and other abnormalities in images. Just look for those features and the diagnosis is made. Unfortunately it is not that easy. According to the Institute of Medicine Committee on Quality of Health Care in America, at least 98,000 people die each year from medical errors.1 Image interpretation accounts for a good number of those errors. In fact, error rates in radiology (both false negatives and false positives) have been estimated to be as high as 30% in some areas. An analysis of the frequency of radiologic image interpretation in the United States a few years back estimated that medical perception and interpretation events occurred at a rate of more than 1 per second.2 There are well over a billion radiology exams conducted every year and that number is growing. There is no doubt that medical imaging plays a vital role in today’s health care system, but like all areas it is not perfect. Errors are made and patient care is impacted on a daily basis. To some extent, that is where the art of interpretation comes into play. Image interpretation is more than just looking for predefined features and calling them out as they are found. The appearance of lesions and other abnormalities change as a function of the technology used to acquire the images, how the images are displayed, and the simple fact that there is so much variation in the basic anatomy within which these targets are embedded that looking for and finding feature x does not always lead to detection and the right diagnostic interpretation. But what makes one radiologist better than another or a given radiologist less error prone on one day than another? How can two experts look at the same image and come up with different interpretations? How can we optimize image presentation in order to optimally educate trainees to ensure the most accurate image interpretation and thus patient care? This is what medical image perceptionists tackle—understanding the art and science of image interpretation to reduce error rates. The Medical Image Perception Society (MIPS; www.mips.ws/) was created to bring together scholars studying the processes of perception and recognition of information in medical images. Physicians, psychologists, statisticians, physicists, and engineers are all members of this growing research community. Every two years, MIPS holds a scientific conference to exchange current research and conduct tutorials and workshops on a wide variety of image perception topics. The meeting promotes medical image perception research and offers students a chance to interact with senior perception researchers. The MIPS XVI Conference was held June 2–5, 2015 in Ghent, Belgium. With nearly 100 attendees, 44 oral presentations, and 14 posters, it was one of the highest attended meetings to date. This special section highlights some of the talks given at the MIPS XVI Conference and illustrates the breadth of approaches that are being taken to understand the image interpretation process, as well as the variety of imaging applications under investigation. All submissions were peer reviewed. The 10 articles cover the core areas that perceptionists are concerned with: detection and discrimination of abnormalities, cognitive and psychophysical processes, perception errors, search patterns, human and ideal observer models, computer-based perception (CAD and CADx), impact of display and ergonomic factors on image perception and performance, role of image processing on image perception and performance, and assessment methodologies. Capitalizing on new imaging technologies, Rousson et al. generated a framework to assess and rank stereo matching methods to optimize breast tomosynthesis and stereo-mammograms. Their long-term goal is to be able to extract depth information from stereoscopic images and develop new visualization techniques that could, for example, measure the depth and radius of a tumor within the breast, detect microcalcifications, and develop CAD systems. Sanchez et al. also investigated breast tomosynthesis, but from the perspective of using model observers. The Hotelling observer was applied to the optimization of linear image reconstruction algorithms in digital breast tomosynthesis, considering information within a specific region-of-interest, and applied to the optimization of algorithms for detectability of microcalcifications. They considered several linear algorithms (e.g., simple back projection, filtered back projection, back-projection filtration). The optimized algorithms were evaluated using phantom data and the method seems to be robust across algorithms and parameters, leading to the generation of algorithms that subjectively appear to be optimized for microcalcification detection. Model observers have been used for a number of years in medical image perception and image quality assessment research and have always been a popular topic at MIPS. Ba et al. evaluated anthropomorphic model observers in 3-D detection tasks for low-contrast CT images. They implemented a novel multislice model observer based on the channelized Hotelling observer (msCHO) with anthropomorphic channels and found that msCHO can be used as a relevant task-based method to efficiently evaluate low-contrast detection for CT and optimize scan protocols to lower dose. Visual search has been a mainstay of medical image perception research since it was first used by Tuddenham in 1962 to study reader error,3 and it is a regular topic at the MIPS conferences. Two papers in this special section illustrate how far we have come with eye tracking and visual search research. Venjakob and Mello-Thoms note that although eye tracking research in conventional (2-D) radiography has been done for over 40 years, the number of eye-tracking studies looking at multislice (3-D) images has been quite low to date. In part, this is due to the fact that volumetric imaging was not common until the advent of digital radiography and computer display of images, but also because eye-tracking methods developed for 2-D images do not readily translate to volumetric imaging. They provide a very nice overview of how these challenges can be addressed in the design of the experiment, opening an entirely new area for image perception research. Auffermann et al. demonstrate that teaching healthcare trainees (physician assistants) a formal search or scan pattern for evaluating lungs improved their ability to identify pulmonary nodules on chest radiographs. All subjects reviewed a set of cases prior to any intervention. One group then received search pattern training and the control group did not. They all then reread the cases. The results demonstrated that teaching a search pattern to trainees improved their ability to identify nodules, and decreased the number of perceptual errors. They suggest that our knowledge of medical image perception may be used to develop new tools for the education of healthcare trainees. Perception studies like this, where readers are required to review the same set of images on more than one occasion as a function of either receiving training or perhaps viewing the images with different displays or image processing applied, also raise the question of whether performance improves simply because they remember the cases from the first session. Evans et al. conducted a unique study to address this question. They tested scene memory using a standard long-term memory paradigm, comparing radiologists with naïve observers on two image sets: chest radiographs and everyday scenes; and radiologists’ memories with immediate versus delayed recognition tests using musculoskeletal radiographs and forest scenes. The radiologists’ memories were better than novices for radiographs but no different for everyday scenes. Radiologists also had better memory for musculoskeletal images than forest scenes, but the effect disappeared over just a few weeks of delay. Extended familiarity (expertise) with an image is not a robust factor for visual memorability. Image quality clearly impacts diagnostic performance as there are fundamental limits to the amount of image noise and degradation the human visual and cognitive systems can deal with. One problem facing radiologists and researchers alike is that different devices often produce images of different quality. Abdolell et al. examined this in the context of percent breast density (PD) and breast cancer risk, noting that it is assumed that visual assessments of PD are comparable between vendors, but this may not be true. They examined the extent to which visual assessments of PD differed between mammograms acquired from two vendors. Overall agreement of the PD assessments was excellent between the two vendors with only a small bias, reassuring us that the human visual system is indeed flexible enough to deal with differences in image appearance at least for certain tasks. Image quality is also the topic off the paper by Razaak and Martini, but with a very different problem—cardiac ultrasound. There exist a variety of state-of-the-art video quality metrics, since video is such a common medium in entertainment, security, and other real-time industries. However, these metrics typically assess perceptual quality, whereas medical videos need to asses diagnostic quality. They developed and tested a diagnostic quality–oriented video quality metric for cardiac ultrasound videos [cardiac ultrasound video quality index (CUQI)], that uses motion and edge information. The metric was evaluated by testing its correlation with subjective scores of medical experts and the results showed high correlation. Preventing errors, improving training methods, and assessing the impact of image quality on performance are just of few of the ways perceptionists aim to improve the interpretation process. In these days of increased pressure to read more images and more complex images in less time,4 finding ways to maintain high levels of diagnostic accuracy is becoming more and more important. Drew et al. tested an image presentation paradigm to assess whether subtle differences can be readily detected when the images are toggled back and forth in the same location. They found that even slightly misaligned pairs of current and prior breast images compared to side-by-side viewing led to a 6 second benefit in time to render a decision as well as a 5% improvement in diagnostic accuracy. This is a clear example of how it is not always necessary to change the image (e.g., process it) to impact detection performance – simply capitalizing on what the human visual does best is often a very effective tool. Finally, the paper by Massanes and Brankov does not deal with perception per se but with a very important MIPS topic nonetheless—how do we assess observer performance? The receiver operating characteristic (ROC) technique is the gold standard for this purpose, but ROC analysis continues to change and be refined. These authors propose a method derived in part from gaming theory, in which multiple rounds of the two-alternative forced choice (AFC) studies (which are often easier to conduct than formal ROC studies) are used to re-estimate image confidence scores/ratings to generate the full ROC curve. Using simulated data and a pilot human study, they found that a full ROC curve can be recovered by using several rounds of 2AFC studies and that the best strategy starts with the first round pairing abnormal versus normal images, followed by rounds using random pairing. The 2AFC study requires less observer time, making it easier to carry out critical observer studies. These are only a few of the exciting and important topics covered in the MIPS XVI Conference. The full set of meeting abstracts can be found on the MIPS website, and updates regarding MIPS XVII to be held in the summer of 2017 can be found there as well. We encourage those interested in gaining a better understanding of how radiologists and other clinicians approach the image interpretation task to attend MIPS XVII. But even for those not involved in image perception research, we believe that it is important to always consider the critical role of the radiologist (pathologist or other healthcare provider) in the interpretation of medical image data and the significant impact this has on patient care. Medical images need to be interpreted because they are not self-explanatory. They vary considerably, anatomical structures camouflage features of clinical interest, lesions often have very low prevalence rates, image quality is affected by bot acquisition and display technologies, and all of these variables impact the decision-making process. These complexities can lead to interpretation errors and have a significant impact on patient care by causing delays or misdiagnoses. We need to understand the amazing capabilities of the human visual system as well as its inherent limitations, and we need to continuously develop new methods and tools to facilitate medical image perception research. ReferencesTo Err is Human: Building a Safer Health System, Committee on Quality of Health Care in America, Institute of Medicine, National Academy of Sciences, Washington DC
(2000). Google Scholar
C. A. Beam et al.,
“The place of medical image perception in 21st-century health care,”
J. Am. Coll. Radiol., 3
(6), 409
–412
(2006). http://dx.doi.org/10.1016/j.jacr.2006.02.029 Google Scholar
W. J. Tuddenham,
“Visual search, image organization, and reader error in roentgen diagnosis. Studies of the psycho-physiology of roentgen image perception,”
Radiol., 78
(5), 694
–704
(1962). http://dx.doi.org/10.1148/78.5.694 Google Scholar
E. A. Krupinski et al.,
“Evaluating RVUs as a measure of workload for use in assessing fatigue,”
Proc. SPIE, 9416 94161A
(2015). http://dx.doi.org/10.1117/12.2082913 Google Scholar
BiographyElizabeth Krupinski, PhD, is a professor and vice chair for research at Emory University in the Department of Radiology and Imaging Science. Her main interests are in medical image perception, assessment of observer performance, medical decision making, and human factors. She has published extensively in these areas, and has presented at conferences nationally and internationally. She is associate director of evaluation for the Arizona Telemedicine Program and serves on the editorial boards of a number of journals in both radiology and telemedicine, and on review panels for NIH, DoD, FDA, and TATRC. She is past chair of the SPIE Medical Imaging Conference, past president of the American Telemedicine Association, and past chair of the Society for Imaging Informatics in Medicine. |
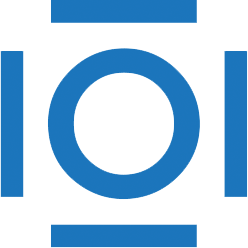
CITATIONS
Cited by 1 scholarly publication.
Medical imaging
Image processing
Visualization
Medicine
Diagnostics
Image quality
Video