|
ACCESS THE FULL ARTICLE
No SPIE Account? Create one
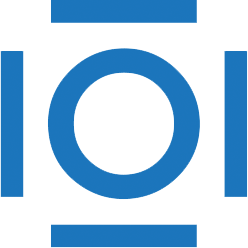
CITATIONS
Cited by 5 scholarly publications.
Gallium nitride
Network architectures
Image segmentation
Super resolution
Convolution
Computer programming
Image quality