|
ACCESS THE FULL ARTICLE
No SPIE Account? Create one
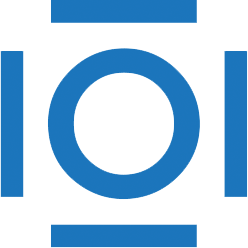
CITATIONS
Cited by 4 scholarly publications.
Binary data
Databases
Image classification
Computer programming
Lithium
Feature extraction
Tolerancing