|
ACCESS THE FULL ARTICLE
No SPIE Account? Create one
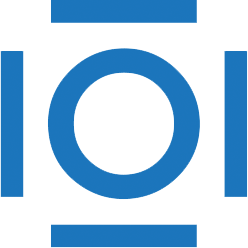
CITATIONS
Cited by 4 scholarly publications.
Machine vision
Associative arrays
Defect detection
Feature extraction
Image segmentation
X-rays
X-ray imaging