|
ACCESS THE FULL ARTICLE
No SPIE Account? Create one
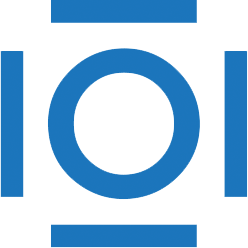
CITATIONS
Cited by 7 scholarly publications.
Databases
Facial recognition systems
Digital imaging
Binary data
Computer vision technology
Machine vision
Chaos