Hyperspectral image (HSI) classification is a hot topic in the field of remote sensing applications. However, due to the high-dimensional and extensive spectral and spatial information of HSIs, effective feature extraction is difficult, which leads to a lower accuracy of HSI classification. In this work, a convolutional neural network (CNN) approach based on a multiscale hybrid 3D-2D-attention mechanism (MHAC) is proposed. Linear discriminant analysis dimension reduction is performed for HSIs, and the central pixel and its adjacent pixels are input into the network as a whole. Based on a multi-scale three-dimensional convolutional neural network (3D CNN) and a two-dimensional convolutional neural network (2D CNN), fine spectral and spatial features are automatically extracted, which improves the network classification capability. At the same time, the channel attention mechanism is embedded in the 2D CNN, and the spatial attention mechanism is embedded in the 3D CNN, which can assign different weights to the retrieved features, effectively utilizing the spatial spectral information to optimize features and improve the feature extraction ability. The experimental results demonstrate that the MHAC achieves an accuracy of 98.72%, 97.60%, and 97.90% on the WHU-Hi dataset and 98.93% on the Indian-Pines dataset based on only 100 samples. The MHAC model proposed in this paper has a good feature extraction ability that effectively reduces the impact of high-dimensional data and small sample problems on classification and improves the accuracy of HSIs classification. |
ACCESS THE FULL ARTICLE
No SPIE Account? Create one
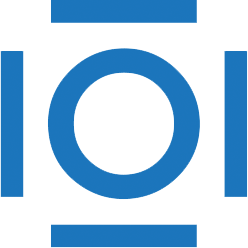
CITATIONS
Cited by 1 scholarly publication.
Feature extraction
Image classification
Education and training
Convolution
3D modeling
Data modeling
Hyperspectral imaging