Domain adaptation is a technology enabling aided target recognition and other algorithms for environments and targets with data or labeled data that is scarce. Recent advances in unsupervised domain adaptation have demonstrated excellent performance but only when the domain shift is relatively small. We proposed targeted adversarial discriminative domain adaptation (T-ADDA), a semi-supervised domain adaptation method that extends the ADDA framework. By providing at least one labeled target image per class, used as a cue to guide the adaption, T-ADDA significantly boosts the performance of ADDA and is applicable to the challenging scenario in which the sets of targets in the source and target domains are not the same. The efficacy of T-ADDA is demonstrated by cross-domain, cross-sensor, and cross-target experiments using the common digits datasets and several aerial image datasets. Results demonstrate an average increase of 15% improvement with T-ADDA over ADDA using just a few labeled images when adapting to a small domain shift and afforded a 60% improvement when adapting to large domain shifts. |
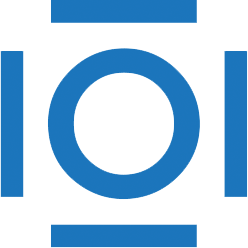
CITATIONS
Cited by 6 scholarly publications.
Computer programming
Feature extraction
Visualization
Data modeling
Gallium nitride
Data centers
Target recognition