Hyperspectral unmixing has become a key technology in hyperspectral image processing. The hyperspectral images contain rich spectral and spatial information. Most algorithms add various constraints and weight factors to make full use of this information and improve the unmixing accuracy. However, the improvement of unmixing accuracy often comes at the cost of large calculations. To solve this problem, we propose the superpixel and low-rank double-sparse regression unmixing algorithm, which can improve unmixing accuracy with lower computational complexity. This algorithm completes the unmixing process by performing two sparse regressions. The result of the first regression is added as a constraint to the second. For the first regression, we perform superpixel segmentation on hyperspectral image to exploit spatial information. To reduce computational complexity, we utilize the mean of each superpixel to represent all pixels in the superpixel for unmixing. In addition, we impose low rankness and sparsity constraints on the mean vector. For the second regression, we impose joint sparsity on the original hyperspectral image. In addition, we employ the reweighting strategy to further enhance the sparsity constraint on every sparse regression. Simulated and real data experiments have proven that the algorithm has improved the unmixing accuracy and unmixing time. |
ACCESS THE FULL ARTICLE
No SPIE Account? Create one
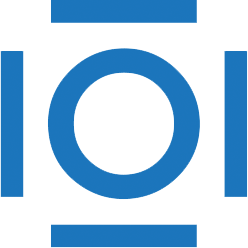
CITATIONS
Cited by 2 scholarly publications.
Signal to noise ratio
Image segmentation
Hyperspectral imaging
Image processing algorithms and systems
Computer simulations
Chemical species
Algorithms