In many remote sensing (RS) applications, haze greatly affects the quality of optical RS images, but we do not always have the conditions to acquire multiple images in the same area for haze removal tasks. Therefore, the research on haze removal from a single RS image is necessary. Previous haze-removal methods introduce various prior knowledge to solve this problem, and thus, the quality of these methods largely depends on the reliability and validity of prior knowledge, which brings various limitations. We propose and validate a deep-learning-based model for haze removal, named haze removal fully convolutional network, to estimate transmission maps and generate corresponding haze-removed images via an atmospheric scattering model. Moreover, we propose an approximate method to produce hazy-and-clear image pairs as a dataset for training and validation. Experiments using this dataset demonstrated that the proposed model achieved the desired results in both visual effect and quantitative measurement. |
ACCESS THE FULL ARTICLE
No SPIE Account? Create one
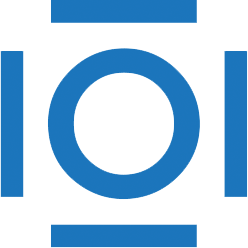
CITATIONS
Cited by 10 scholarly publications.
Air contamination
Remote sensing
Image transmission
Atmospheric modeling
Image processing
Image quality
Image restoration