|
ACCESS THE FULL ARTICLE
No SPIE Account? Create one
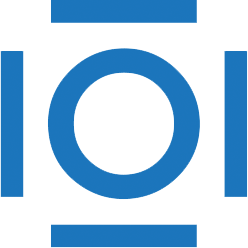
CITATIONS
Cited by 1 scholarly publication.
Feature extraction
Mammography
Digital mammography
Breast cancer
Image segmentation
Digital imaging
Tissues