|
ACCESS THE FULL ARTICLE
No SPIE Account? Create one
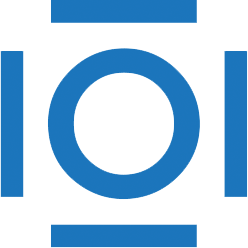
CITATIONS
Cited by 2 scholarly publications.
Image segmentation
Image filtering
Convolution
Image classification
Linear filtering
Statistical analysis
Binary data