|
ACCESS THE FULL ARTICLE
No SPIE Account? Create one
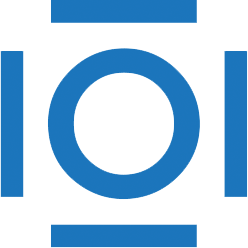
CITATIONS
Cited by 1 scholarly publication.
Image compression
JPEG2000
Linear filtering
Computer programming
Discrete wavelet transforms
Transform theory
Wavelets