|
ACCESS THE FULL ARTICLE
No SPIE Account? Create one
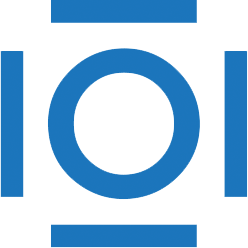
CITATIONS
Cited by 4 scholarly publications.
Evolutionary algorithms
Image classification
Remote sensing
Earth observing sensors
Landsat
Data modeling
Roads