|
ACCESS THE FULL ARTICLE
No SPIE Account? Create one
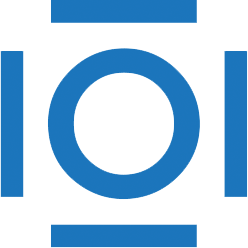
CITATIONS
Cited by 19 scholarly publications.
Data modeling
Hyperspectral simulation
Scene simulation
Hyperspectral imaging
Translucency
Factor analysis
Image analysis