|
ACCESS THE FULL ARTICLE
No SPIE Account? Create one
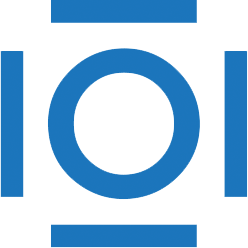
CITATIONS
Cited by 2 scholarly publications.
Image segmentation
Image processing algorithms and systems
Error analysis
Image analysis
Mathematical modeling
Phase modulation
Image processing