|
ACCESS THE FULL ARTICLE
No SPIE Account? Create one
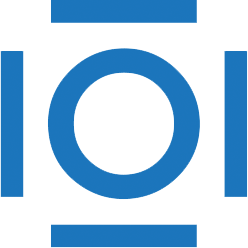
CITATIONS
Cited by 1 scholarly publication.
Image segmentation
Neural networks
Computed tomography
Image processing algorithms and systems
Lung
Databases
Evolutionary algorithms